The best customer interactions require some amount of reading between the lines.
When agents can identify and address any underlying, unstated concerns that the customer has, they can provide a much better solution.
Predictive customer service is a way to do that on a much larger scale.
What is predictive customer service?
Predictive customer service is a business strategy that uses data analytics, machine learning, and AI to anticipate customer needs and issues before they occur.
It has a lot in common with proactive customer service. Both methods are about looking for ways to provide support preemptively and providing solutions in advance, ideally before a customer has even noticed that there might be an issue.
The main difference between the two approaches is the technology used.
A company can provide proactive customer service with a few clever automations. In contrast, predictive customer service relies on:
Advanced data analytics to process data about customer interactions, purchase history, browsing habits, feedback, and so on to predict their future actions or needs.
Machine learning models that can forecast behaviors like churn risk, product interest, or potential service issues.
Generative AI models that suggest or send out automated messages like personalized offers or troubleshooting steps.
These predictions can also be used to trigger alerts for the customer support or success team so they can provide help before predicted issues become major problems.
Here are a few example use cases of predictive customer service in action:
A telecom company might identify customers likely to experience service disruptions and proactively offer solutions or compensations before the customer contacts support.
An online retailer could predict when a customer might be ready to reorder a frequently purchased item and send a personalized reminder or discount offer. Amazon does this — it’ll highlight products that you purchase often and ask if it’s time for a refill.
A healthcare provider might anticipate which patients are likely to miss appointments and proactively send reminders or offer alternative scheduling options.
These might sound like small tweaks to the customer experience, but they can lead to huge results for businesses if implemented correctly.
Does predictive customer service require AI?
Predictive analytics doesn’t require AI, although AI features can make the process a lot better.
A predictive analytics approach without AI might use:
Statistical techniques like regression analysis, time series analysis, and decision trees. These methods analyze historical data to identify trends and predict future outcomes.
Rule-based models based on algorithms that apply a predefined set of rules to make a prediction.
In the simplest form, a business might use linear regression to predict future sales based on historical sales data and factors like seasonality or marketing spend. A credit scoring system could also use a rule-based model to predict credit risk based on variables like income and credit.
These are useful applications for predictive customer service if training an AI model is too time-consuming or resource-intensive.
An AI model can help you level this up, though, because it can analyze large and complex datasets, learn from them, and improve predictions over time. They’re also especially useful for areas requiring image recognition or natural language processing, like customer feedback, since it takes more effort to process large amounts of unstructured data.
The benefits of predictive customer service
Using predictive analytics in customer service provides many of the same (potential) benefits that using generative AI does:
Higher customer retention: Predictive analytics can be useful for predicting (and mitigating) churn risks. This is one of the highest-value areas to invest in as a business — any time you can reach out and retain customers who might otherwise leave, it’s a big win.
Improved brand loyalty: Unsurprisingly, customers prefer brands they can connect with and that provide a personalized experience. In fact, leaders in personalization have a 1.5 times higher customer loyalty rate than their peers. Since predictive customer service is based on analyzing the behavior of that particular customer, using it effectively (in a way that improves their experience) can have a massive impact.
Higher customer satisfaction: Addressing potential issues before they become problems is a great way to surprise and delight your customers. It isn’t about setting up a chatbot that sends a repetitive message; rather, it’s about using information that directly pinpoints the specific customer's needs. When your smart fridge identifies that your water filter’s efficiency is decreasing and you get an email reminding you to order a new one, it’s a good experience.
Increased efficiency: Proactive problem-solving should reduce the volume of inbound questions so your support team can focus on more complex issues (or on creating more of those delightful experiences in their interactions).
The three key challenges of implementing predictive customer service
Predictive customer service is an approach that’s been used for some time. That said, getting started with it doesn’t come without challenges.
Using high-quality data
Predictive customer service is a data-driven approach.
How effective it will be depends on how accurate, comprehensive, and consistent the data you’re working with is.
Many companies struggle with data quality. Inconsistent, incomplete, or siloed data can undermine the accuracy of any model you develop. The results are misguided predictions, a lot of wasted time, and customer service interventions that have no impact, at best, or that cause harm by incorrectly predicting customer needs.
Before you start, the data you’re working with has to be clean, standardized, and — ideally — integrated across all systems.
Developing and maintaining a complex predictive model
Even if you don’t choose to train an AI model for this (which might be a wasted opportunity, considering how easily accessible they are now), developing a complex, rule-based algorithm will still be time-consuming.
The best predictive models can consider multiple factors while analyzing a large volume of customer data. You also need to hit the right balance of complexity — it has to be complex enough to capture important nuance without being so complex that it’s difficult to maintain or interpret.
As customer behaviors and market conditions evolve, you’ll probably need to evaluate the model's performance and adapt it regularly.
It’s generally a good idea to start with a simpler model and increase its sophistication as the team uses it more. In the first instance, you only need to predict one or two specific behaviors and test out a handful of ways of responding to them. Then you can slowly scale from there.
Addressing ethical concerns and privacy issues
Processing customer data at this scale can also raise ethical concerns. While predictive customer service can be great at highlighting opportunities to personalize, it’s much harder to analyze data in aggregate and comply with data privacy laws.
That means you’ll need to invest in securing customer data through encryption, the maximum level of anonymization possible, and access controls.
Transparency is also key. It’s important to communicate to customers how their data is being used and obtain explicit consent where necessary.
Best practices for predictive customer service
Almost 90% of consumers find proactive customer service a pleasant surprise or a positive experience.
It’s much easier to delight customers when they aren’t expecting it.
The opportunities for predictive customer service can be really effective. Here are a few best practices and examples of companies already doing it today.
Focus on high-impact use cases
Prioritizing use cases that offer a high return on investment (ROI) and are feasible with your current resources ensures that your efforts are impactful and sustainable.
You’ll reach enough customers to observe their impact. An obvious example is credit card fraud: Almost every credit card provider now has fraud detection systems so they can react quickly.
Visa, which manages around 300 billion transactions per year, has implemented an AI model that analyzes 500 attributes per transaction and gives them a score. This has helped them save a whopping $40 billion in fraud over the course of one year, which is more than double what they could do with predictive analytics without AI.
This is the type of implementation that’s a no-brainer for a business — it has a massive impact on customers (and their loyalty) and on the business as a whole. It also makes it easy to invest in further opportunities opened up by AI.
Maintain customer trust and provide value
As mentioned above, privacy concerns can be one of the barriers to implementing predictive customer service.
Most customers have two key priorities:
The interaction should provide value for them. For example, if a company is providing personalized recommendations based on browsing behavior, that recommendation is much more powerful when it resonates with what the customer is looking for.
They need clear communication about data usage and a commitment to protecting customer privacy.
Caesar’s Palace in Las Vegas has been using predictive models for a few years now. They deliver tailored offers with high perceived value but low variable costs, like free room upgrades or special promotions. These offers are carefully calculated to ensure they are profitable and don’t cannibalize revenue from trips customers would have taken anyway, and their customers don’t mind because they also benefit from the upgrades and promotions.
Measure impact
As with most new approaches to customer service, taking the time to establish KPIs and monitor the outcome of each initiative is the best way to ensure the desired impact is there.
Predictive models can be unreliable, or your method could lead to a different result than expected. Identifying your success criteria in advance makes it easy for you to respond to both scenarios.
Netflix is the perfect example of a company that’s built much of its success with predictive models. Its recommendation model influences 80% of the total content watched on Netflix — and they’ve been doing this for years, long before generative AI became as widely used as it is today.
They’ve achieved that by continually refining their algorithm based on user engagement metrics. That type of ongoing maintenance and long-term fine-tuning is the best way to achieve long-lasting success.
Creating delightful customer experiences
Creating delightful customer experiences is a lofty goal, but as customer expectations rise, it’s more vital than ever.
Predictive customer service can play a crucial role in helping you achieve this. Anticipating customer needs and delivering personalized, proactive solutions that resonate with individual customers greatly impacts their loyalty.
The main challenge is implementing AI (and other algorithms) thoughtfully and in a way that puts customers first.
That is ultimately the most effective strategy for long-term success.
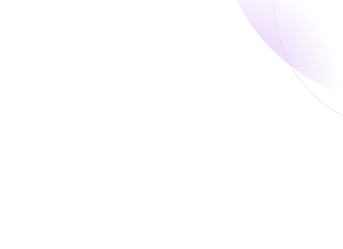