AI’s ability to deliver a great customer experience hinges on one key factor: data quality.
Without high-quality data, even the most advanced AI tools will struggle to provide accurate, helpful responses. Your customers will walk away frustrated and unsatisfied, and you’ll miss out on the potential value of your AI.
As a customer support leader, understanding how to improve data quality will help you provide a more effective and reliable AI support experience.
How does AI use data to help provide support?
There are many benefits to using AI in customer support, but the bottom line is that all AI relies heavily on data to function, “learn,” and deliver accurate responses.
But what exactly does that data look like?
AI-powered support tools typically draw from a range of data sources, including:
Knowledge base articles: These articles contain detailed information about products, services, and common customer issues. AI uses these articles to give customers clear and accurate answers to common questions.
Past customer conversations: Conversation logs from previous customer interactions provide context for AI tools to understand how similar queries were handled before and what solutions were provided.
Web content: Publicly available content, like FAQs or product descriptions on your website, can be ingested by AI to provide customers with relevant information.
The more relevant and structured the data, the better customer experience your AI can deliver.
The importance of data quality in AI-powered customer service
It’s not an overstatement to say that the quality of your data will make or break your AI’s success.
If the data feeding your AI tools is incomplete, outdated, or inaccurate, it can lead to misinformation, frustrated customers, and, ultimately, a decline in trust in your support system (and brand).
Imagine someone whose only exposure to history was from watching alternate history TV shows. Now say that same person gets invited to a pub trivia night where all the categories are real history.
Suffice it to say that they’re in for a rough night.
If your AI is trained on inaccurate or outdated info, it’s going to face similar challenges when fielding questions from your customers.
So, what makes data high quality? Here are four things to keep in mind.
Accuracy: The data AI relies on must be correct and error-free. If your knowledge base or past conversations contain incorrect information, your AI will deliver those same mistakes to customers.
Completeness: Incomplete data sets result in AI systems that can’t provide comprehensive responses. If key information is missing, the AI may have to "guess," increasing the likelihood of poor results.
Consistency: Data should be consistent across all systems. Conflicting information in different data sources can confuse AI and lead to inconsistent customer experiences.
Relevance: Your AI tools need data that is both relevant to customer inquiries and up to date. Outdated or irrelevant content will make your AI appear uninformed and unreliable.
When AI relies on subpar data, it leads to incorrect or "hallucinated" answers, which is when AI generates responses which sound plausible but are factually incorrect.
This frustrates customers and creates issues that could have easily been avoided — potentially including opening you up to legal ramifications, like when Air Canada was held responsible for their chatbot’s misinformation.
How data quality can affect AI performance
You might be using some of the best AI tools out there, but your data still has to be good or your customers will suffer. To illustrate the importance of quality data, let’s look at three examples of how AI data quality can make or break AI performance.
Example 1: Microsoft’s Tay AI chatbot
In 2016, Microsoft released an AI chatbot called “Tay,” designed to interact with humans on Twitter to develop conversational intelligence. In other words, Microsoft relied on the potential Wild West of social media interactions to feed Tay data.
Within 24 hours, Tay started posting racist and misogynistic content due to online campaigns to feed it that type of information. The result? Microsoft shut down Tay after only a day of it being released.
The support lesson: Use good judgment when feeding your AI data and proactively review the data before it’s ingested.
Example 2: Amazon’s internal AI recruiting tool
In 2014, Amazon started using an AI tool to help them recruit faster by analyzing resumes and giving the top five candidates for the role. In 2015, they realized the tool was showing gender bias by not rating female candidates nearly as high as men in more technical roles.
The issue was that Amazon’s AI models were trained to observe patterns in resumes over a period of 10 years — and most applicants were male.
As reported by Reuters, “Amazon's system taught itself that male candidates were preferable. It penalized resumes that included the word ‘women's,’ as in ‘women's chess club captain.’ And it downgraded graduates of two all-women's colleges.”
After several update attempts, Amazon ultimately disbanded the team creating and updating the model because there was low confidence that the AI tool wouldn’t find a way to do it again.
The support lesson: Details matter. Not only do you need to give your AI tool access to accurate data, but the data has to be relevant and monitored for bias to make sure the results help your customers and support your organization’s goals.
Example 3: H&M’s chatbot guards against incorrect answers
H&M’s support chatbot on their website is designed to give quick answers to common questions and help find products on the H&M website. Because of this specific use case, the bot is trained to handle only a small amount of data.
This guards against hallucinated answers but also means the bot may not have access to what a customer might ask — or how they might ask it. To get around this, H&M has built in a polite response explaining the bot isn’t understanding and suggests some questions to ask based on the initial customer question.
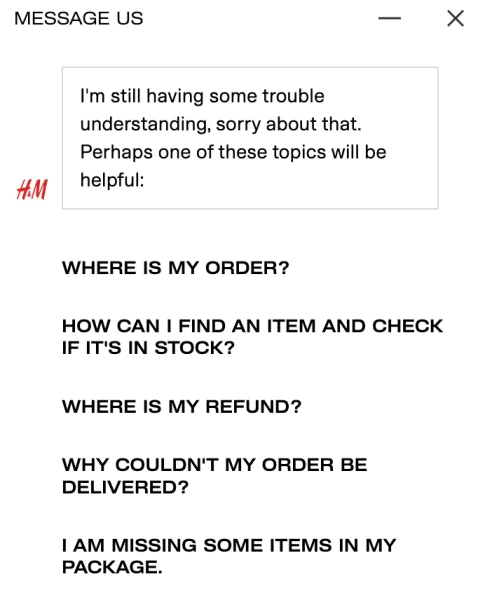
The support lesson: It’s important to have a good support experience even when your AI tool is forced to have access to only a limited amount of data.
Best practices for maintaining data quality
Now that we’ve covered the importance of AI data quality, what are some best practices for maintaining the data your AI is using? Luckily, many of the same practices you’re already using to create great customer experiences also apply to maintaining your data for AI.
1. Regular data audits
Conduct regular audits of your data sources, especially your knowledge base and customer service documentation.
Look for data sources that haven’t been updated in a while. It’s easy for help center articles to become outdated or irrelevant as your products and services evolve.
By setting up a schedule to review and update your documentation, you ensure that your AI tool stays equipped with the most current information.
2. Create clear data ownership
One of the easiest ways to ensure your data remains accurate and up to date is to assign clear ownership over data quality.
Designate responsibility to specific team members or departments for maintaining different data sources, such as your knowledge base, training materials, and customer support tech stack. This ensures accountability and streamlines the process of keeping data in top shape so you’re not doing it all yourself.
3. Train AI models with diverse data sets
The more data you provide your AI, the better customer experience it’ll provide — but only if that data is diverse and actually represents real-world customer interactions.
For example, Cars Commerce (my current employer) serves both car dealers and consumers. If the AI support assistant was only trained on dealer customer issues, it wouldn’t serve consumers well. If we’re going to implement effective AI, we need to make sure the system is trained on both dealer and consumer information and that it has clear ways to distinguish between which data is relevant for any given customer interaction.
Make sure your AI is trained on a broad set of data that covers the variety of questions and concerns your customers might have. This will reduce the chances of the AI falling short when faced with complex or uncommon questions.
4. Leverage AI data quality tools
AI data quality tools can significantly reduce the time it takes to maintain the data being used for your AI.
Tools like Great Expectations or Talend are designed to verify if the data being fed into AI systems adheres to predefined quality metrics, such as accuracy, completeness, and validity. They can also help ensure customer data is accurate and free of errors before being used in customer support AI responses.
Investing in these tools allows you to take a proactive approach to improving your data quality. AI data quality tools can identify gaps, inconsistencies, or outdated information, allowing you to clean your data before it becomes a problem.
5. Integrate feedback loops
Let’s face it: You’re not going to catch everything before it gets out of the proverbial AI door.
That’s where customer feedback comes in. Customer feedback is invaluable for improving both AI performance and data quality. Whenever a customer flags an incorrect or irrelevant response, that information should be reviewed and used to improve your AI model’s training.
Whoever is reviewing that data can use the feedback to determine if an adjustment needs to be made to the algorithm, if an update needs to be made to the bot language, or if it’s a simple data quality issue. Use this feedback to improve the underlying data and prevent future errors.
How to ensure your AI tool isn’t giving hallucinated answers
One of the biggest risks of poor-quality data is AI-generated hallucinations. Even when your data quality is great, hallucinations can still happen.
So how can you reduce the chances of your AI tools supplying customers with hallucinated answers? Here are some proactive steps you can take to catch them early and ensure your AI is delivering accurate information.
1. Validate responses with human oversight
Although AI can handle a large volume of customer inquiries, human oversight is still essential. Implement workflows where human agents review AI responses, particularly for complex or high-stakes issues.
Think of this as quality assurance for AI. In fact, if you have a QA program for your human agents, you should also consider reviewing AI-handled conversations.
Designate a couple of team members to both review past AI responses for quality and monitor high-impact issues in real time. By validating these responses, you can identify potential errors early and adjust the data accordingly.
2. Use fallback mechanisms and a higher confidence threshold
You’ll never be able to provide every resource to an AI tool no matter how hard you try, so you need a backup.
Many AI tools include a confidence threshold: If the AI’s confidence in its answer isn’t high enough, it should trigger an alternative response.
A good AI system includes fallback mechanisms for when it can’t help, such as offering to pull in a human agent or pointing the customer to additional resources. This reduces the risk of providing misleading answers and improves the overall customer experience.
3. Monitor AI performance metrics
Monitor metrics such as response accuracy, first-contact resolution, and customer satisfaction (CSAT) when AI is involved. These metrics will give you a sense of how well your AI tool is performing and whether data quality issues are causing a drop in service levels. Regular monitoring allows you to quickly identify and correct any problems with the data being used.
4. Implement continuous learning for AI
AI models are not static; they should be constantly learning from new interactions and refining their approaches. Many tools like ChatGPT can learn from both successful interactions and mistakes, so the best way to help your AI continually learn is by feeding it new information.
Your AI model should be plugged in so that it’s constantly being trained by your other tools — your knowledge base, your customer conversations, your CRM, and more. This continuous learning helps to ensure that data quality issues are identified and corrected in real-time, improving the accuracy of future responses.
Great data quality is essential for great AI support
An accurate AI support experience improves customer satisfaction and enhances your support team's performance.
Whether you’re using an entire AI help desk, agent assist tools, or simply an AI assistant chatbot, high-quality data is the foundation of any successful customer-first, AI support strategy.
By auditing data regularly, assigning ownership, training AI with diverse datasets, and validating responses, you can ensure that your AI tools always provide value, not confusion.
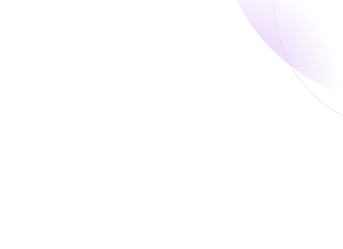