The buzz around AI isn’t dying down just yet. Nearly 80% of businesses consider automation a critical part of their customer experience strategy.
AI and chatbots can be applied thoughtfully to enhance your customer experience and enable your team to focus on creating more impact.
But what does “thoughtfully” look like in practice?
It starts with choosing the right technology and tool for your business.
Here’s how to differentiate between chatbots and conversational AI. We’ll explore their differences and optimal use cases, and we’ll provide real-life examples and tips for choosing your ideal solution.
Understanding the difference between chatbots and conversational AI
Chatbots and conversational AI are terms that tend to be used interchangeably, and while there can be an overlap, they aren’t exactly the same thing.
What is a chatbot?
A chatbot is a computer program designed to simulate conversations with human users via a text-based interface. Chatbots analyze user messages and generate responses by following predetermined conversation flows or using artificial intelligence.
The concept of chatbots dates back to the 1960s when the first chatbot, Eliza, was created. Eliza is a simple computer program that acts as a psychotherapist – analyzing a user’s input and applying a set of rules to return a relevant response.
Since then, chatbots have evolved significantly. Today, businesses employ them to automate customer interactions, deliver around-the-clock customer service, and impress customers with rapid response times.
What is conversational AI?
Conversational AI is the technology that allows machines to comprehend human language and engage in dialogue with users. It’s the underlying mechanism behind many chatbots, enabling them to replicate human language patterns.
To identify user intents and produce human-like responses, conversational AI relies on two key components:
Natural language processing (NLP) enables computers and machines to understand human language and grasp nuances like intent, tone, and style. It’s also used to generate responses almost indistinguishable from how humans speak.
Machine learning (ML) then uses statistical algorithms to analyze data — like previous chat interactions — to learn and improve the program’s output over time with minimal human involvement.
So, what's the difference?
Chatbots are the front-end interface through which customers can interact with machines (e.g., a support platform).
Conversational AI is the back-end technology capable of understanding human speech and generating responses that simulate real conversation.
What about generative AI?
Generative AI is another AI technology that focuses on creating original content, be it images, music, text, or even code. It is trained on large language models (LLMs) which help generative AI-powered apps create original content based on human-supplied prompts.
While there is overlap between generative and conversational AI, each has a different focus. Generative AI is about using large amounts of data to generate something that is new, while conversational AI is about understanding human language and facilitating realistic dialogue between people and machines.
Many chatbots — like ChatGPT created by OpenAI — use a combination of conversational and generative AI technologies to power their user experiences.
Rule-based chatbots vs. conversational AI chatbots
Chatbots can be rule-based, leading users through pre-defined flows or powered by AI, autonomously replying to inquiries using AI-driven logic.
Rule-based chatbots follow a predetermined set of rules or workflows and respond to customer queries with scripted responses. Replies are triggered based on specific keywords, user attributes, or other criteria.
Here's an example of a traditional chatbot flow that guides users through different conversation paths depending on whether they require assistance with product usage, wish to report a bug, or have another question.
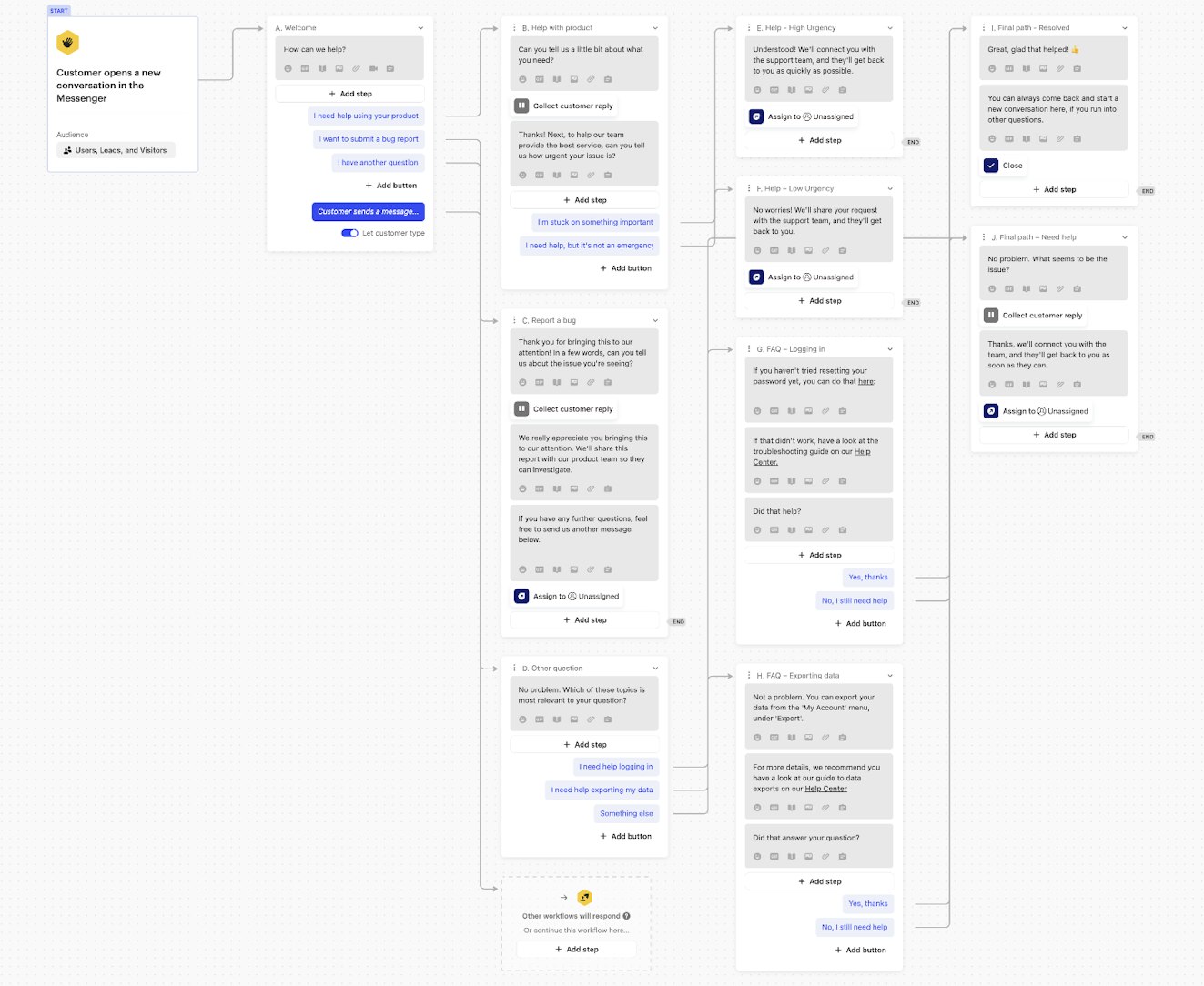
On the other hand, AI chatbots can understand the context and generate original responses, often based on information sourced from your knowledge base or past customer interactions.
They can adjust their responses to better suit user emotions and intentions. For example, if a customer is frustrated due to extended delivery times, a conversational AI bot can offer an apology along with the estimated shipping time frame.
What exactly sets them apart?
The difference between rule-based and AI chatbots is a little like the difference between making a cup of coffee using an espresso machine vs. using a Keurig-type machine.
With an espresso machine, you can meticulously measure out the beans, grind them just before using them, froth the whole milk, and control each step of the process to achieve the perfect result — a luxuriously creamy latte.
That’s what building a rule-based chatbot is like. It lets you carefully design predetermined rules and responses to guide customer conversations. This hands-on approach gives you complete control over the final outcome, although it also means you need to design the full decision tree every interaction will go through.
Conversational AI bots are more similar to choosing a K-cup and pressing a button on a Keurig. While it’s quicker and more convenient, the end result is affected by many factors beyond your immediate control.
You can still adjust the settings and influence the final product, but the outcome will be less predictable and consistent. That might work in your favor in some cases. Say a customer responds to a chatbot prompt in an unexpected way. A rule-based chatbot will be stumped, whereas an AI chatbot may be able to adapt to respond more naturally.
Rule-based chatbot | AI-powered chatbot |
---|---|
Predetermined conversation flows, covering a limited set of pre-defined scenarios | Non-linear and dynamic conversations, simulating human dialogue |
Uses rule-based linear interactions triggered by specific keywords or attributes | Uses natural language processing and generative AI |
Pre-scripted responses to specific common questions | Original responses based on context adapted to user emotions and intents |
Scope limited to pre-configured flows and questions | Continuous learning with responses improving with each interaction |
Chatbot use cases in customer support
Rule-based chatbots and those powered by AI might appear similar to end-users, but they serve different purposes, rely on different technologies, and are not interchangeable. Each of them has its pros and cons. That’s why choosing the right technology for your customer service team’s use case makes all the difference.
Use cases for rule-based chatbots:
Rule-based chatbots excel at automating straightforward customer service requests, frequently asked questions, and data collection. Common examples include:
Welcoming site visitors and gathering their details for lead qualification and sales outreach.
Informing customers about their order status and estimated shipping times and addressing other requests that don’t require deep analysis.
Collecting data for bug reports, such as the user’s email address, browser version, and operating system.
Providing instructions for resolving common issues like how to complete a return or reset a password.
Use cases for AI bots:
AI chatbots can handle more complex requests, anticipate customer needs, and provide assistance through genuine human-like interactions. Popular use cases for conversational and generative AI-powered chatbots include:
Addressing queries that can be answered with your knowledge base content or information from previous customer interactions.
Providing multi-language support by offering relevant answers in the requester’s preferred language.
Offering personalized recommendations by analyzing customer order history, website views, and other attributes.
Handling abstract questions and requests, such as creating itineraries, triaging requests, and assisting with troubleshooting.
Real-world conversational chatbot examples
The share of companies employing chatbots and conversational AI is growing at unprecedented speed. By 2027, about 25% of all businesses will have chatbots as their primary customer service channel.
Given the trend, here are a few inspiring real-life examples of chatbots in customer service with different applications, benefits, and outcomes.
AI chatbot examples in customer service
1. Zack from Zapiet
Zapiet created their bot Zack to provide 24/7 support coverage.
The bot answers various user questions based on Zapiet’s extensive knowledge base content, covering topics like account settings, billing issues, basic troubleshooting, and integration questions.
Zack also shares the source article for more information, driving more visitors to the help center resources. The Zapiet team uses CSAT surveys to assess the bot’s performance.
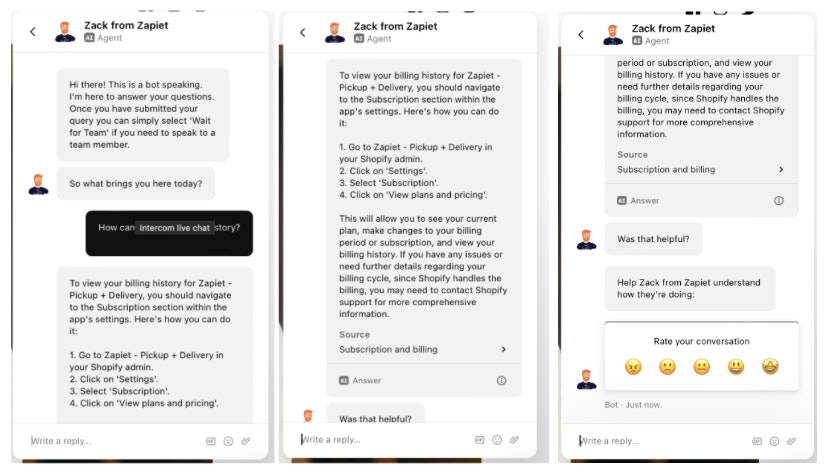
2. Wealthsimple’s conversational support bot
Wealthsimple, the Canadian company that helps consumers manage their finances and investments, has a super helpful chatbot that uses conversational AI.
The Wealthsimple bot pulls from its extensive help center, but it doesn’t just share links. It uses their knowledge base to provide assistance in a human-like chat conversation. As users ask the bot questions, it takes the help center’s content and transforms it into a chat-friendly, conversational format.
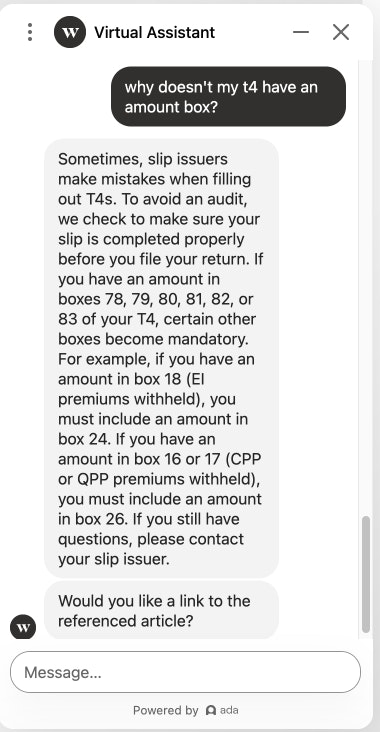
One great thing about the Wealthsimple bot is that after sharing what it believes is the correct answer, it asks if the customer wants to see the link to the article it’s pulling information from. This is a thoughtful touch because it makes it easy for customers to get more context if desired without overwhelming them with irrelevant information.
Examples of rule-based chatbots in customer service
1. Delta Air Lines virtual assistant
Delta’s chatbot helps the company respond to basic customer questions. It replies with pre-scripted responses addressing popular questions about flight changes, cancellations, mileage, profile details, baggage, and eCredits.
It also gathers additional information to provide the most relevant responses. For instance, following the flight cancellation flow, the bot confirms the cancellation reason and can provide immediate assistance or share the refund policy first.
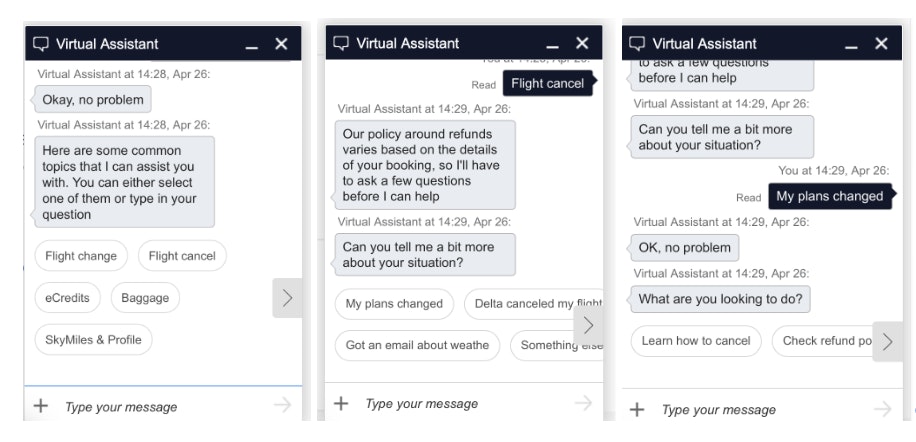
2. H&M's friendly chatbot
H&M offers a chatbot on their website, too. Instead of guiding customers through pre-defined flows, the bot triggers responses based on keywords in the user's message. It prompts users to rephrase their questions if it fails to identify any preset keywords.
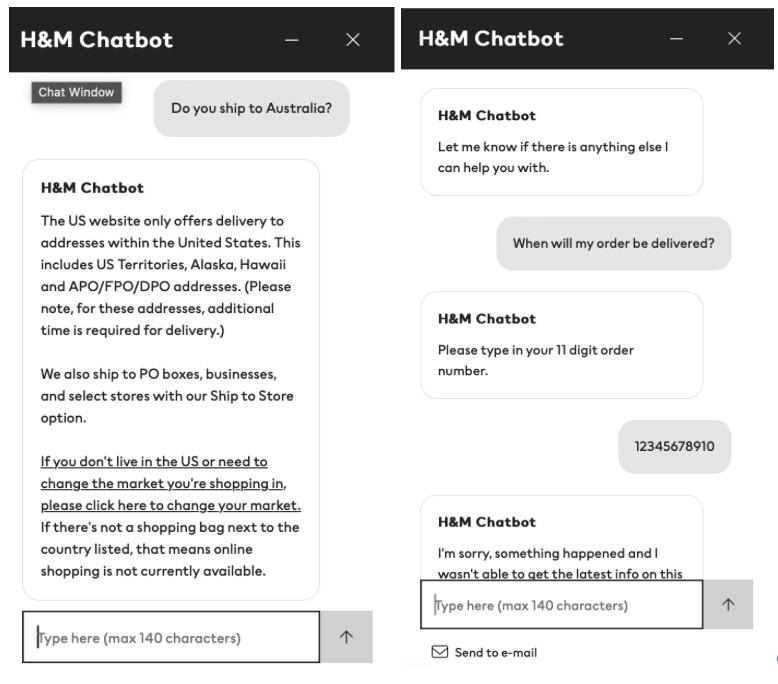
It’s helpful for most common questions, such as shipping zones and order or refund status. However, if you need help with something more advanced, you’ll still need to reach out to their human agents via email.
How to choose the right type of chatbot for your business
The most important factor in determining which type of chatbot you implement is the nature of the customer inquiries you want to automate.
When to choose a rule-based chatbot
A rule-based chatbot can be the perfect choice if your support team mostly deals with straightforward and repetitive customer inquiries.
In some industries, most interactions are standardized, transactional, and require quick responses. For example:
In retail, I've found that up to 80% of support inquiries come from standard questions about shipping time frames, cancellations, and return policies. Rule-based chatbots are great at efficiently addressing those types of queries without human intervention.
In the hospitality sector, hotels can streamline reservations, menu-related inquiries, and room service requests using rule-based chatbots with pre-configured flows.
When to use a bot powered by AI technology
Conversational AI bots are preferred for questions that may not be satisfied by a scripted response but can still be addressed using information from prior customer conversations and knowledge base content.
They’re also great in industries such as healthcare, education, and travel, where you may need to offer more complex and personalized support.
One caution is that conversational AI bots require substantial processing power, and the cost per ticket is typically higher. This means that you’ll need to keep cost in mind when assessing ROI.
Combining both technologies
In some cases, you might choose to implement both.
A rule-based chatbot can efficiently gather basic customer information using pre-defined flows, while an AI bot can deliver personalized experiences based on that data.
In industries like SaaS, where customer inquiries often demand deep analysis and log review, leveraging both rule-based and AI-powered chatbots can be a huge help.
Implementing chatbots successfully
The success of any tool that interacts with your customers hinges on aligning technology with your business objectives and customer expectations.
Whether you opt for the precision of rule-based chatbots or the adaptability of conversational AI, the key is to choose the right tool for the job. The goal is a mixture of increasing customer satisfaction and efficiency for your team and maximizing ROI.
The more automation you implement, the more crucial it is to find that balance to ensure that you’re always delivering experiences that truly delight your customers.
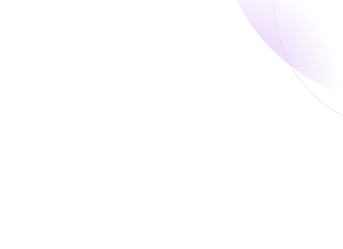